Smart Data Research UK’s director, Joe Cuddeford reflects on the Government’s AI Opportunities Action Plan
The government’s AI Opportunities Action Plan, unveiled at the start of the year, outlines a bold ambition to transform the UK into a global leader in artificial intelligence. Amid eye-catching proposals – for supercomputers, AI Growth Zones and a National Data Library – is one crucial recommendation that risks being overlooked: the call to “actively incentivise and reward researchers and industry to curate and unlock private datasets”.
This matters. If AI continues to be built on proprietary data controlled by a handful of dominant tech companies, barriers to entry will prevent smaller players and independent researchers from harnessing the full potential of AI for public benefit.
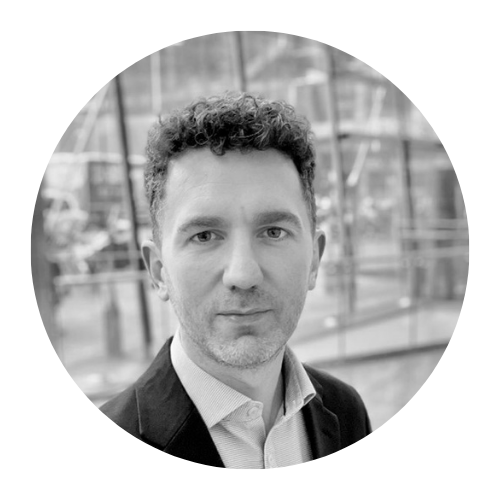
At Smart Data Research UK, we’re working with companies, researchers and the public to create secure, ethical ways to access private sector data for research that benefits society. Part of this involves exploring how AI can help derive valuable insights from large, unstructured datasets. But as AI evolves rapidly, new challenges and opportunities emerge for research data infrastructure.
More than computing power
It’s often said that the three key ingredients of AI’s progress are compute, talent and data. The AI Opportunities Action Plan rightly addresses all three, but data may be the trickiest part to get right1.
The first high-profile LLMs, like ChatGPT, were trained primarily on web-scraped datasets such as Common Crawl. That’s no longer enough. As models become more sophisticated, and as we look for practical applications of AI technology beyond chatbots, different data is needed. Elon Musk’s xAI, for example, trains its LLM ‘Grok’ on Twitter/X user data, taking advantage of data that’s unavailable to competitors2.
While general-purpose generative AI models are good at creating content and summarising complex information, some of the most impactful practical applications may emerge from more specialised ‘narrow’ AI systems. Models that focus on specific, well-defined tasks – such as energy optimisation or healthcare – often outperform general-purpose models at those tasks. This makes intuitive sense – AI trained on high-quality, specific data, built with input from subject-matter experts who understand the system being modelled, will return more useful results.
This trend highlights the growing data divide. Startups, public bodies, and independent researchers lack access to the data they need to participate in AI innovation. Vast amounts of data generated by ordinary citizens – our financial transactions, shopping habits, and social media interactions – are largely inaccessible for public-interest research. The International AI Safety Report, compiled by 96 AI experts, highlights the systemic risks arising from market concentration among a few key players.
Making private data work for public good
At Smart Data Research UK, we’re investing £30 million in new national data services that make private-sector data safely available for research. These services will provide new opportunities to study economic behaviour, public health, sustainability, and social trends.
Our Imagery Data Service (Imago), for example, is exploring how AI can extract insights from satellite imagery. The Geographic Data Service is working with retailers to map economic activity across the UK. The Smart Data Donation Service is pioneering ways for individuals to volunteer their own digital footprint data for scientific study. The Financial Data Service will offer secure access to deidentified financial data.
It’s early days, but all these initiatives have security, ethics, and public trust at their core. The aim is to create a trusted ecosystem where valuable data can be used responsibly for public good.
Rethinking data infrastructure for the AI age
Much of today’s data-intensive research relies on Trusted Research Environments (TREs) – secure settings which were developed to enable statistical analysis of sensitive data. But as AI-driven research brings new demands, these systems need to evolve.
We’re working with partners such as DARE UK to explore new models for secure research data environments. Challenges include:
- Developing standards for federated analysis (allowing queries to be sent to data across physically separated TREs, rather than moving raw data into one place).
- Rethinking output auditing, ensuring that AI models trained on sensitive datasets don’t inadvertently expose private information.
- Connecting high-performance computing with TREs, to enable AI approaches that demand greater processing power than these environments were originally designed to support.
While AI presents challenges for research infrastructure, it also brings new opportunities. It can help streamline workflows and unlock insights from complex, unstructured datasets.
What it hasn’t replaced, however, is the ongoing need for good data management. Transforming raw, messy data into research-ready datasets remains an essential but often underinvested step in the process. If we want AI to deliver real impact, we need to get the foundations right.
Broadening the talent pool
To create AI systems that benefit society, we need multidisciplinary teams that combine technical expertise with deep insights into social, ethical, and methodological considerations. Social scientists, for example, can bring crucial insight into data bias, human behaviour, ethics and socioeconomic systems. All of these are essential to making AI work in the real world.
Our data services are designed to support multidisciplinary collaborations. For instance, our Imago data service aims to both ‘meet researchers where they are’ by developing new AI methods to translate complex satellite imagery into research-ready data, while also provide training and outreach to grow the UK’s research capability.
But to achieve a step change in public benefit from AI, we may need to fund new kinds of support to researchers who don’t see themselves as ‘AI experts’, so they can confidently engage in AI development.
Fixing the incentives for data sharing
So, how should government respond to the challenge posed by the AI Opportunities Plan: to actively incentivise private-sector data sharing?
First the good news – many businesses already recognise their broader social and environmental responsibilities, beyond financial returns. As companies seek to demonstrate their commitment to ethical practices, responsible data-sharing can become part of how they deliver positive social impact. Our pilot projects have demonstrated the feasibility and value of voluntary data sharing partnerships and our new data services build upon this.
But there are limits to corporate goodwill. In his book, The Coming Wave, Mustafa Suleyman, co-founder of DeepMind, writes about his attempts to rethink the corporate mandate within Google (spoiler: it was a frustrating experience). Profit has historically been – and will continue to be – a powerful engine of innovation and progress. In some sectors, like social media, we have longstanding gaps in access to data for researchers.
Effective government intervention can correct for market failures. I saw this during my time at the Geospatial Commission, which led the creation of the National Underground Assets Register. Until recently, the UK had no single map of underground pipes and cables, despite the economic damage and threat to life caused by accidents. Convincing all utility companies and telcos to share data, even when it was in their collective interests, has required an Act of Parliament.
Change on the horizon
Governments are acting. The EU’s Digital Services Act requires large online platforms to provide vetted researchers with access to platform data to study systemic risks like misinformation and electoral manipulation. This sets an important precedent: companies can be required to share data responsibly for the public interest. In the UK, Ofcom is consulting on current constraints on data sharing for research, to assess how greater access might be achieved.
To accelerate this shift, the UK could introduce a Data Contribution Obligation. Such a framework would require companies above a certain size to share anonymised datasets with accredited researchers via approved schemes3. This wouldn’t be easy, given the complex regulatory landscape, competitive markets and the international nature of data flows – but it should be addressed as an urgent part of the next round of tech regulation.
At the same time, we can empower citizens to take control of their own data. Initiatives like our Smart Data Donation Service offers one model. It will enable individuals to exercise their GDPR rights and contribute their data to research in a way that is transparent, ethical, and beneficial to society. The Data (Access and Use) Bill aims to help consumers and businesses to securely share their data with authorised third parties. This should foster innovation and competition.
Seizing the AI moment
The UK has an opportunity to shape AI development in a way that is fair, open, and beneficial for all. At Smart Data Research UK, we hope to be part of this by building secure data infrastructure, adapting research environments for AI, and fostering multidisciplinary innovation.
But achieving the vision of the AI Opportunities Plan will require bold action and leadership. If we want AI to serve social progress, we need a system that ensures data is shared responsibly.
Endnotes
- For more on the delivery challenges of research data infrastructure, I recommend Ben Goldacre’s recent paper on how to implement a National Data Library. ↩︎
- In many ways, the data access landscape has become more uneven. For instance, the Twitter API, once a cornerstone of internet studies, was drastically restricted in 2023, cutting off access for many researchers. ↩︎
- The Digital Economy Act has helped advance this for public sector data and highlights that legislative reform must be backed by investment in effective data infrastructures like ADR UK. ↩︎